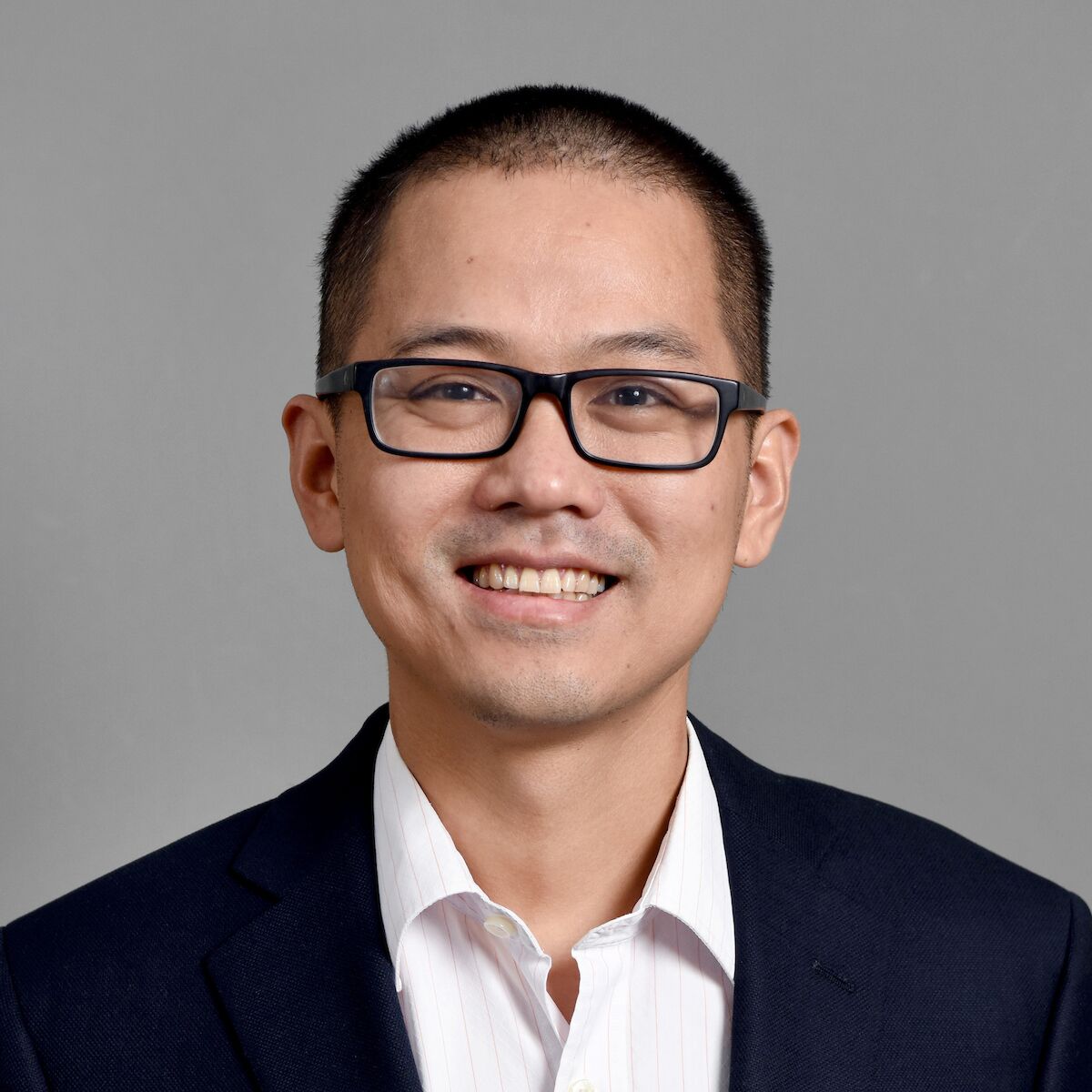
Event Details |
---|
Thursday, December 9, 2021 Talk:
1:30–2:30 p.m., Zoom
Reception:
N/A, N/A
|
Hanghang Tong, Ph.D.
Associate Professor, University of Illinois at Urbana-ChampaignAbstract
Network (i.e., graph) mining plays a pivotal role in many high-impact application domains. State-of-the-art offers a wealth of sophisticated theories and algorithms, primarily focusing on answering who or what type question. On the other hand, the why or how question of network mining has not been well studied. For example, how can we ensure network mining is fair? How do mining results relate to the input graph topology? Why does the mining algorithm `think’ a transaction looks suspicious?
In this talk, I will present our work on addressing individual fairness on graph mining. First, we present a generic definition of individual fairness for graph mining which naturally leads to a quantitative measure of the potential bias in graph mining results. Second, we propose three mutually complementary algorithmic frameworks to mitigate the proposed individual bias measure, namely debiasing the input graph, debiasing the mining model and debiasing the mining results. Each algorithmic framework is formulated from the optimization perspective, using effective and efficient solvers, which are applicable to multiple graph mining tasks. Third, accommodating individual fairness is likely to change the original graph mining results without the fairness consideration. We develop an upper bound to characterize the cost (i.e., the difference between the graph mining results with and without the fairness consideration). Toward the end of my talk, I will also introduce some other recent work on addressing the why & how question of network mining, and share my thoughts about the future work.
Speaker Bio
Hanghang Tong is currently an associate professor at Department of Computer Science at University of Illinois at Urbana-Champaign. Before that he was an associate professor at School of Computing, Informatics, and Decision Systems Engineering (CIDSE), Arizona State University. He received his M.Sc. and Ph.D. degrees from Carnegie Mellon University in 2008 and 2009, both in Machine Learning. His research interest is in large scale data mining for graphs and multimedia. He has received several awards, including ACM distinguished member (2020), ICDM Tao Li award (2019), SDM/IBM Early Career Data Mining Research award (2018), NSF CAREER award (2017), ICDM 10-Year Highest Impact Paper award (2015), and several best paper awards. He is the Editor-in-Chief of SIGKDD Explorations (ACM), and an associate editor of ACM Computing Surveys (ACM) and Knowledge and Information Systems (Springer); and has served as a program committee member in data mining, database and artificial intelligence venues (e.g., SIGKDD, SIGMOD, AAAI, WWW, CIKM, etc.). He is a fellow of IEEE.